Climate change has affected the timing of many ecological events, from tree leaf out to bird migration. These changes in phenology can cause mismatched timing of life history events for multiple species, for example asynchrony in pollinator activity and flower blooming. However, testing whether phenological shifts occur is a research challenge because of limited historical data on the timing of life history events. By combining field experiments and AI-based detection of individual body characteristics, this research offers a new approach for determining the age and activity period of an important crop pollinator. Image analysis using convolutional neural networks (CNN) is very efficient for classifying images without the need for manual feature extraction. As part of this project you would develop a deep-learning automated wing identification system (DeepAWIS) using a reference image library. You would then train a CNN to detect and quantify the proportion of individual wings that are degraded. This work provides the foundation for computational analysis of museum specimens and future field-validation. Your work would put this research on the path towards new methods for investigating phenological shifts in the age structure of an important pollinator. There is also the opportunity to be included as a co-author on subsequent publications.
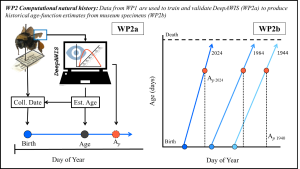
Computational natural history to back cast individual age. We will use the empirical data from field work to develop a convoluted neural-network (CNN) for wing image analysis. This CNN (DeepAWIS) will detect the degree of wing wear to generate an estimate of individual age and time to/from age of peak function (Ap). We will apply DeepAWIS to wing images taken from B. terrestris specimens housed in the Biological museum of Lund University (WP2a). Collection information allows us to generate data historic lifespan trajectories over time, for example from 1940 (light blue) to current day (dark blue). These lifespan trajectories provide estimates of three age parameters: (1) the day of year (DOY) for emergence (blue point), (2) age at capture (black point), and (3) Ap (red point). This novel approach allows us to track whether the timing of age parameters have changed over time (WP2b).
Duration 30-60 cr.
Contact: Charlie Nicholson (he/him), PhD